Apr 14, 2017
Chinese AI Wins $290k against Six Poker Pros
By RTR Dennis
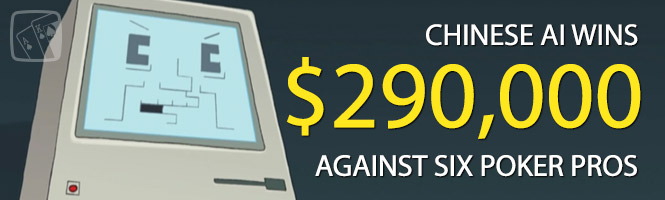
Much has been made about artificial intelligence programs (AIs) beating poker players. And we can add one more story to this trend because a Chinese AI has beaten six poker pros out of $290,000 (£230,000).
Dubbed Lengpudashi, the AI faced off against poker pros over a 5-day span. The humans were led by Yue Du, who won $800,586 last year in a $5,000 WSOP Texas hold'em event. Du's 'Team Dragon' consisted of a mix of players and engineers who used their poker/AI knowledge to counter Lengpudashi.
Texas hold'em was often thought to be a tough game for AIs to beat because, in contrast to chess and Go, it features imperfect information. However, AIs have advanced considerably to the point where they can use complicated betting strategies, and counteract their opponents' bluffs. And Lengpudashi put this on full display by defeating Du and his team of poker pros by $290k.
Third Time that AIs have Defeated Humans
While certainly notable, Lengpudashi's victory isn't even close to the most-famous AI win over poker pros. Earlier this year, Libratus defeated four elite poker pros – Dong Kim, Daniel MacAulay, Jimmy Chou, and Jason Les – to the tune of $1,766,250.
Developed by Carnegie Melon University, Libratus' win was seen as a landmark in the AI community. In 2015, Carnegie Melon's AI, Claudico, was soundly defeated by almost $800,000 against Les, Kim, Bjorn Li, and Doug Polk. Few expected the latest version, Libratus, to come back and win so easily.
Libratus was developed by a team of researchers at Carnegie Melon, led by computer professor Tuomas Sandholm and PhD student Noam Brown.
"People think that bluffing is very human," said Brown, "It turns out that's not true. A computer can learn from experience, that if it has a weak hand and it bluffs, it can make more money."
Another less-heralded contest took place between the University of Alberta's AI, DeepStack, and a group of 11 poker pros. Each player was asked to play 3,000 hands or more over four weeks against DeepStack. The result included DeepStack triumphing over the humans after 44,000 hands.
Computer scientist Michael Bowling, who led the University of Alberta researchers in this project, said that poker has long been a challenge to his profession. However, it looks like the AI community has taken a big step towards overcoming this obstacle.
“It is the quintessential game of imperfect information in the sense that the players don’t have the same information or share the same perspective while they’re playing,” said Bowling.
Another Milestone in Artificial Intelligence
Victories by Lengpudashi, DeepStack, and Libratus confirm that AIs have passed humans in the game of imperfect information. And this is just the latest landmark in what's been an ongoing process since the 1980s.
It all began in the early 1980s, when developers began trying to defeat chess. It didn't take long to defeat a human chess player, but it would be a long time before the AI community solved chess.
This came on May 11, 1997, when the IBM computer Deep Blue defeated world chess champion Garry Kasparov in six games. Deep Blue won two games, Kasparov won 1, and they had three draws.
Afterward, IBM's website noted that the competition 'was important computer science, pushing forward the ability of computers to handle the kinds of complex calculations needed to help discover new medical drugs; do the broad financial modeling needed to identify trends and do risk analysis; handle large database searches; and perform massive calculations needed in many fields of science.'
In the late 2000s, the focus became how AIs could win at limit hold'em. This task was accomplished quickly because limit hold'em has fixed betting limits, unlike the no-limit version. Now, over three decades after the journey of game problem-solving began, researchers have beaten another game in no-limit hold'em.
How are AIs Better than Human Poker Players?
According to Bowling, the University of Alberta bot uses a technique called continual re-solving to improve its poker game and defeat humans.
'Each situation itself is a mini poker game,' he explained. 'Instead of solving one big poker game, it solves millions of these little poker games, each one helping the system to refine its intuition of how the game of poker works. And this intuition is the fuel behind how DeepStack plays the full game.'
No matter how difficult a decision is, DeepStack can react at an average of three seconds. This is in great contrast to top professional players, who sometimes take minutes to make decisions in live games. Being able to solve millions of 'poker mini games' in seconds gives the AIs a big advantage over their human counterparts.
It also helps that fatigue is never a factor. In fact, an AI can continue solving problems and improving away from a competition, whereas a human player eventually needs to rest, or they begin operating at less than optimal level.